The SCCN lab studies how humans recognize other people and acquire knowledge about them, as well as disorders of social cognition, using a combination of behavioral studies, computational models, and neuroimaging. Research is organized along the following main focus areas:
Perception
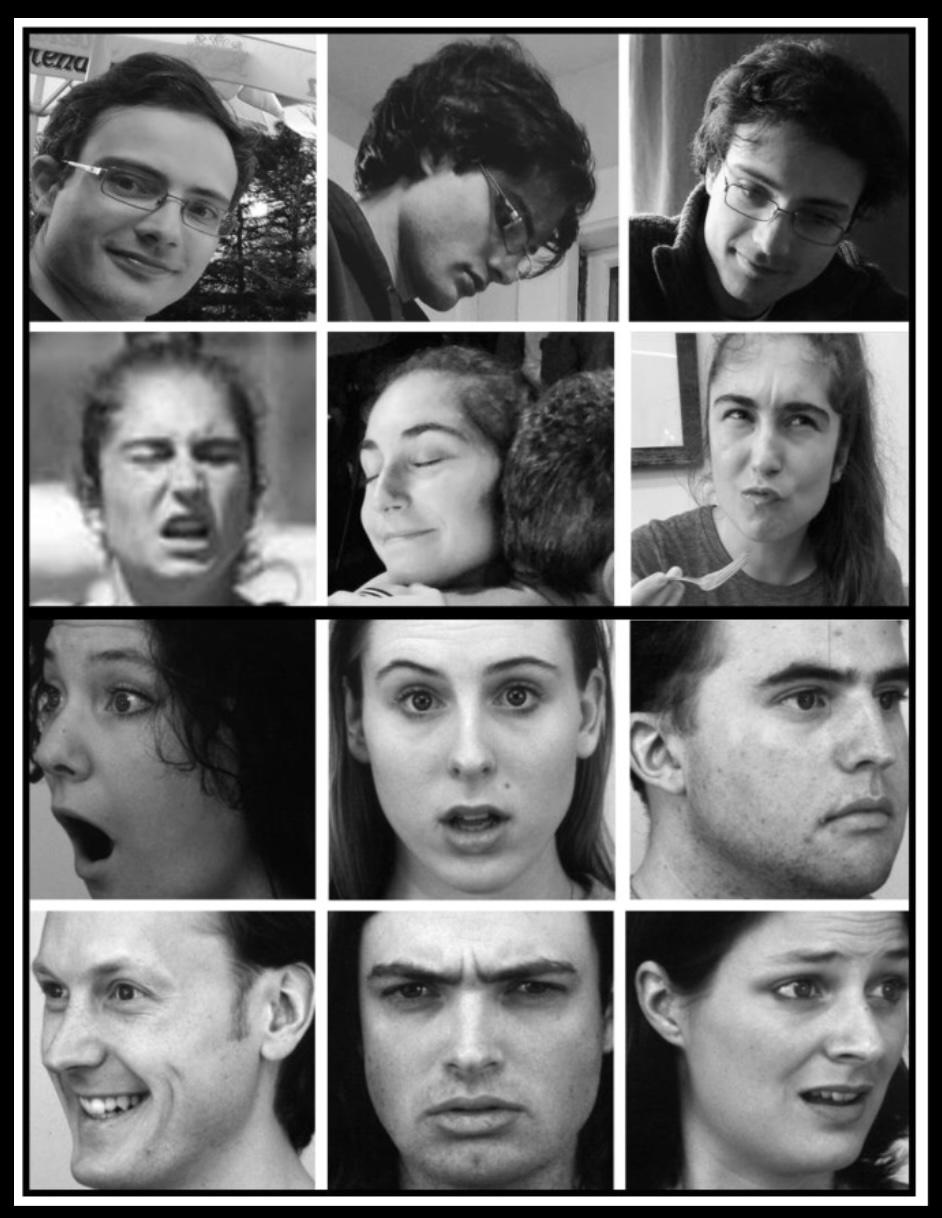
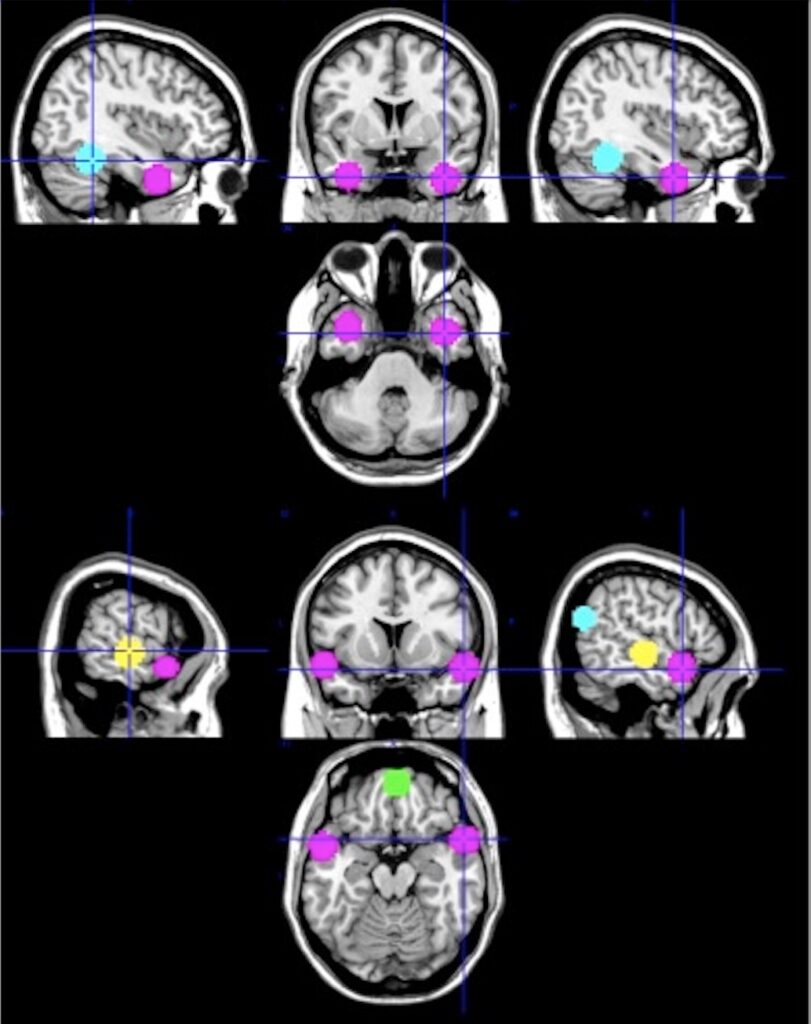
How do we use sensory inputs to make sense of the world around us? The SCCN lab’s work has focused on the perception of social stimuli like faces, asking how the brain represents information that supports the recognition of a person’s identity and their facial expressions. Using neural data including functional MRI and intracortical EEG in combination with multivariate analyses and deep neural networks, we found that identity and expressions are not processed by separate neural pathways as previously thought, but instead share common neural substrates. More recently, we are extending our work to the investigation of the representations of actions and events, asking how perception enables us to build causal representations of the environment that enable us to engage in counterfactual reasoning and planning.
Anzellotti, S., Fairhall, S. L., & Caramazza, A. (2014). Decoding representations of face identity that are tolerant to rotation. Cerebral cortex, 24(8), 1988-1995.
Anzellotti, S., & Caramazza, A. (2017). Multimodal representations of person identity individuated with fMRI. Cortex, 89, 85-97.
Schwartz, E., O’Nell, K., Saxe, R., & Anzellotti, S. (2023). Challenging the classical view: recognition of identity and expression as integrated processes. Brain Sciences, 13(2), 296.
Schwartz, E., Alreja, A., Richardson, R. M., Ghuman, A., & Anzellotti, S. (2023). Intracranial electroencephalography and deep neural networks reveal shared substrates for representations of face identity and expressions. Journal of Neuroscience, 43(23), 4291-4303.
Social Cognition
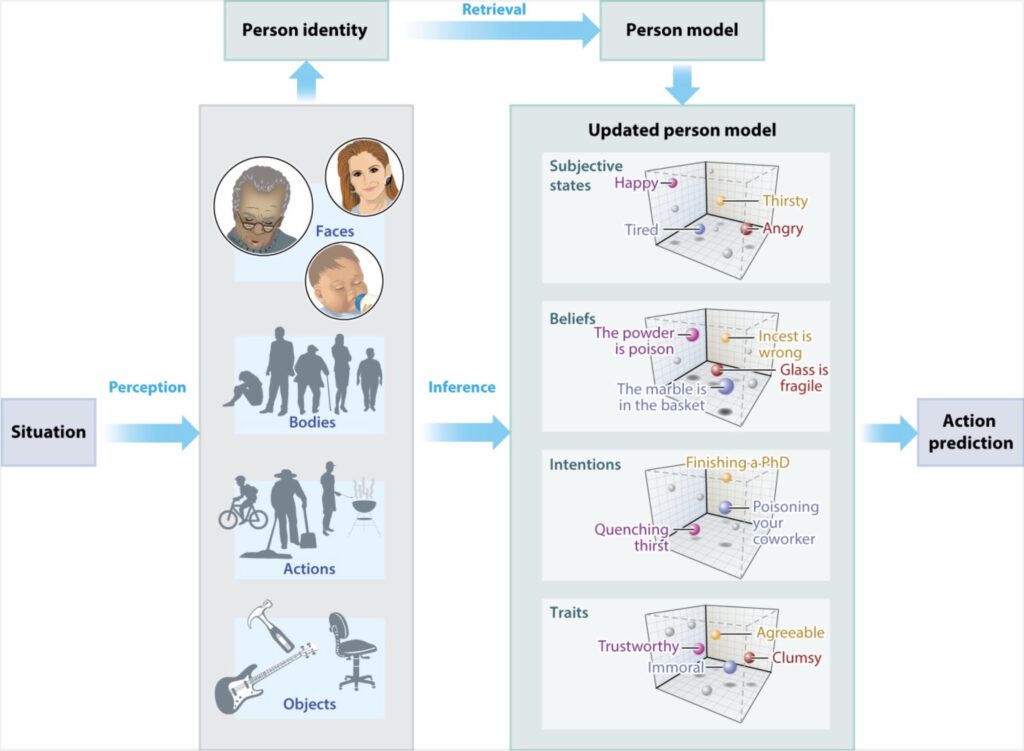
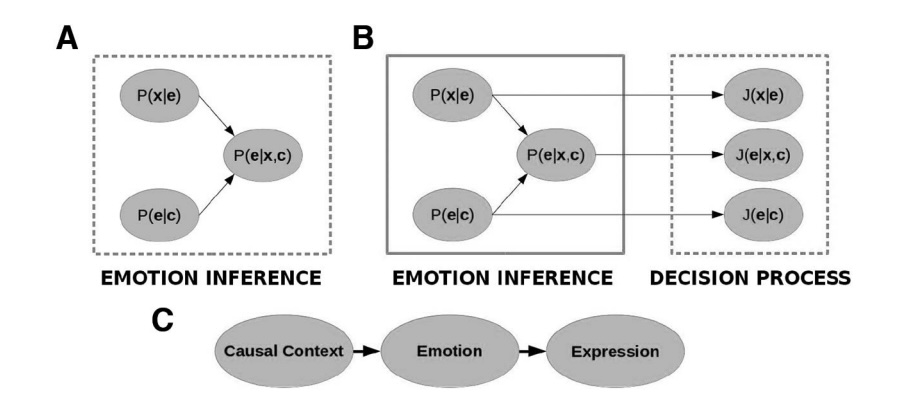
Our lives are shaped by interactions with other people. Understanding others requires bridging the gap from perception to person knowledge: inferring others’ goals, beliefs, emotions and traits. Person knowledge is a particularly interesting case study of how humans make sense of the world. Not only because of the importance it plays for our lives, but also because people are unusually complex entities: their behavior follows patterns that results from the interplay of environmental factors, the person’s traits, and dynamically changing internal states. Understanding other people is among the hardest cognitive challenges humans face, investigating how we can solve it is essential for the study human intelligence. In ongoing work, the SCCN lab is using computational models to study how we make inferences about others from observing their behavior, with the goal to scale from the understanding of simple agents in virtual environments (grid worlds) to increasingly more realistic scenarios that involve agents with dynamic internal states and complex environments.
Anzellotti, S., & Young, L. L. (2020). The acquisition of person knowledge. Annual Review of Psychology, 71(1), 613-634.
Anzellotti, S., Houlihan, S. D., Liburd Jr, S., & Saxe, R. (2021). Leveraging facial expressions and contextual information to investigate opaque representations of emotions. Emotion, 21(1), 96.
Kim, M. J., Mende-Siedlecki, P., Anzellotti, S., & Young, L. (2021). Theory of mind following the violation of strong and weak prior beliefs. Cerebral Cortex, 31(2), 884-898.
Kim, M., Young, L., & Anzellotti, S. (2022). Exploring the representational structure of trait knowledge using perceived similarity judgments. Social Cognition, 40(6), 549-579.
Neurodevelopmental disorders
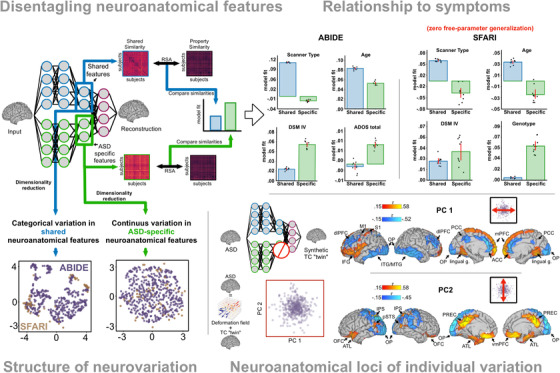
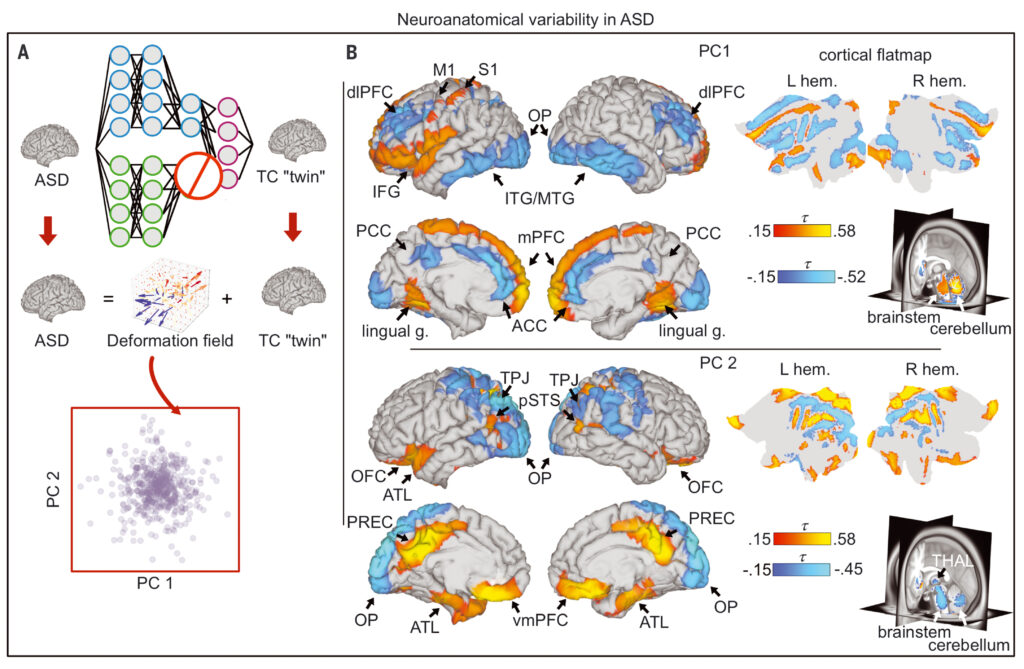
Neurodevelopmental disorders that affect social cognition (such as autism) affect millions of individuals. Understanding their causes and developing interventions has been challenging. One key difficulty concerns the high degree of heterogeneity among individuals diagnosed with the same disorder. The SCCN lab is investigating the structure of this heterogeneity, with the hope that this can lead to more effective personalized interventions. In recent work, the lab has demonstrated that separating disorder-related individual variation in brain measures from individual variation in common with the general population with artificial neural networks can reveal otherwise hidden relationships between brain measures and behavioral symptoms.
Aglinskas, A., Hartshorne, J. K., & Anzellotti, S. (2022). Contrastive machine learning reveals the structure of neuroanatomical variation within autism. Science, 376(6597), 1070-1074.
Aglinskas, A., & Anzellotti, S. (2022). Precision psychiatry requires disentangling disorder‐specific variation: The case of ASD. Clinical and Translational Medicine, 12(10).
Methods development
A central part of the SCCN laboratory’s activity is the development of novel methods to address the scientific questions we are interested in. Over the years, this has led to the development of new approaches for the study of multivariate connectivity between brain regions, for the disentanglement of disorder-related information in neural measures, and for improved denoising of neuroimaging data.
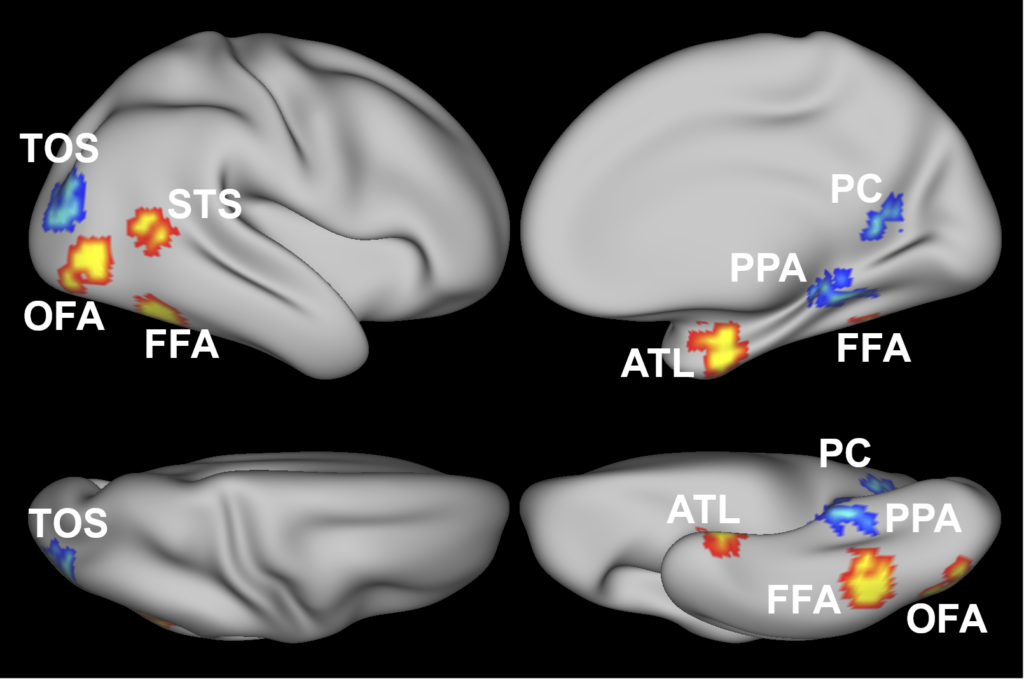
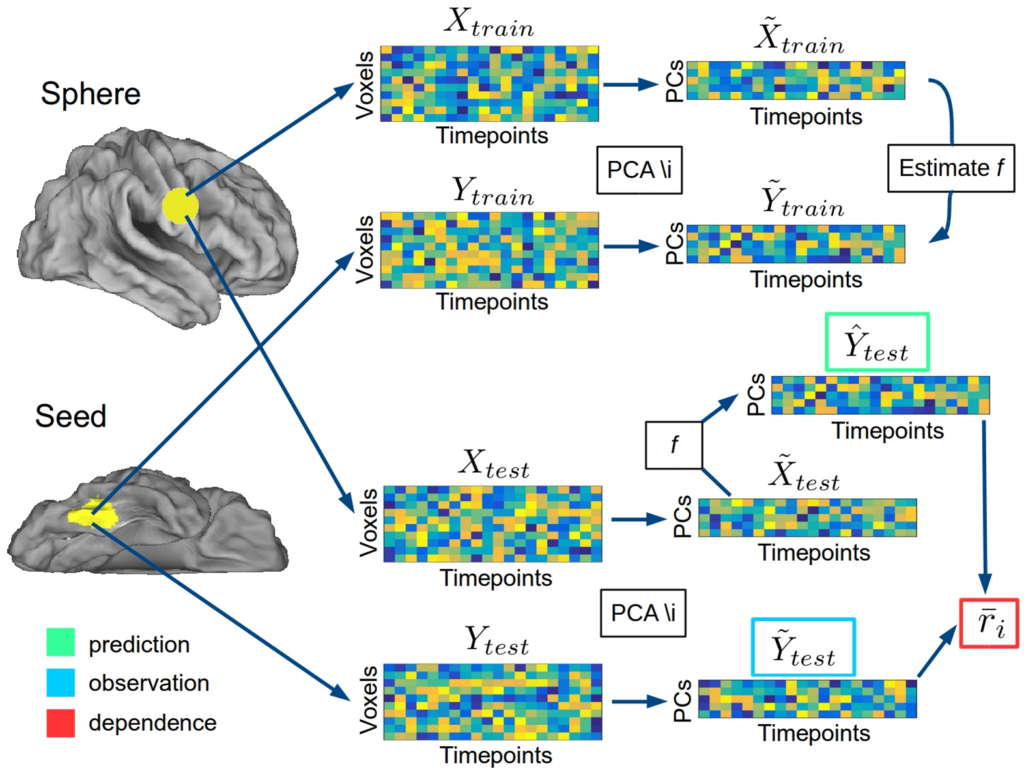
Anzellotti, S., Caramazza, A., & Saxe, R. (2017). Multivariate pattern dependence. PLoS computational biology, 13(11), e1005799.
Li, Y., Saxe, R., & Anzellotti, S. (2019). Intersubject MVPD: Empirical comparison of fMRI denoising methods for connectivity analysis. PloS one, 14(9), e0222914.
Fang, M., Poskanzer, C., & Anzellotti, S. (2022). Pymvpd: A toolbox for multivariate pattern dependence. Frontiers in neuroinformatics, 16, 835772.
Fang, M., Aglinskas, A., Li, Y., & Anzellotti, S. (2023). Angular gyrus responses show joint statistical dependence with brain regions selective for different categories. Journal of Neuroscience, 43(15), 2756-2766.
Aglinskas, A., Hartshorne, J. K., & Anzellotti, S. (2022). Contrastive machine learning reveals the structure of neuroanatomical variation within autism. Science, 376(6597), 1070-1074.
Zhu, Y., Aglinskas, A., & Anzellotti, S. (2023). DeepCor: Denoising fMRI Data with Contrastive Autoencoders. bioRxiv, 2023-10.